前言
"Paper 整理"系列只是試著將paper中的概念,或是將別人對paper的見解整理起來,幫助自己消化及日後複習。都會附上出處,如有侵權或錯誤煩請email告知:jason.eed05@gmail.com
話說這是我第一篇讀的論文,實在嚴重消化困難阿......。
論文連結
Abstract
BERT - Bidirectional Encoder Representations from Transformers
簡言之,BERT是個可以將輸入資訊(詞語、句子)中的特徵(feature)、表徵(representation)提取出來的model。(BERT中的R便是從此而來)
然後將表徵用於下游訓練(論文說通常只需在model下面多加just one additional output layer),便可在所需任務上取得出色成果。
Introduction
Pre-training
假設已經有人在某一任務 $T_A$上,運用自己的 dataset $D_A$ 將其設計的model A訓練的很好,
日後其他人遇到相似性質的任務 $T_B$ 時,便不用再自己另外設計一個model B,
而是可以直接用model A 的架構,並只在(前面)後面的 layer 做新增、修改,甚至在訓練時model A部分的參數都可以沿用前人以龐大dataset $D_A$ 訓練好的參數而不是random initialize 。
(優點是當任務 $T_B$ 的dataset較少時,model很難訓練好;但是獲得了以 $D_A$ 訓練的參數,有時表現會優很多)
屬於 transfer learning??(不確定)
Fine-tuning
目前應用 pre-trained language representations在下游任務的策略主要有兩種:feature-based and fine-tuning。
- Feature-based: 把原本pre-trained的語言模型的輸出作為"額外特徵"送給應用於新任務的model,幫助學習。訓練過程中提供feature的model 權重固定。
- Fine-tuning: 在原本pre-trained的語言模型的基礎上增加少量神經網路以完成新任務。然後訓練時語言模型的參數會變動。
BERT
Transformer 架構。左半部為encoder,右半部為decoder。
(source: https://pic3.zhimg.com/80/v2-b530e1f8d16d3b6b0fc9ce9bfaa7748a_hd.jpg)
可能會在另寫一篇詳細介紹Transformer以及Attention。不過關於transformer 的 encoder & decoder 之間差異,這裡先直接引用論文:
We note that in the literature the bidirectional Transformer is often referred to as a “Transformer encoder” while the left-context-only version is referred to as a “Transformer decoder” since it can be used for text generation.
較早的模型 OpenAI GPT 可能是為專注在 "text generation" 而使用的transformer 是decoder 部分。而這造成了OpenAI GPT model 只能獲得前文資訊而不是像 BERT 可以同時獲得前後文資訊。
來看一下較早的模型ELMo,雖然號稱是雙向LM,但是實際上只是將兩個unidirectional RNN在最後一層拼接起來罷了。
BERT 與其他 pre-training model 架構的比較
(source: https://pic3.zhimg.com/v2-f946f9bccb723606f813cf21e83b6a2a_r.jpg)
Input Representation
"The input embeddings is the sum of the token embeddings, the segmentation embeddings and the position embeddings."
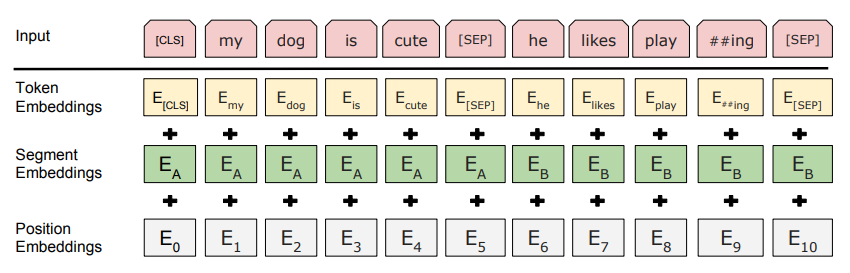
(source: https://pic2.zhimg.com/v2-11505b394299037e999d12997e9d1789_r.jpg)
- Token Embeddings 是詞向量(使用WordPiece embeddings),第一個單詞是CLS標誌,可以用於之後的分類任務
- Segment Embeddings 用來區別兩種句子。因為預訓練除 Masked LM 還有以兩個句子為輸入的 Next sentence prediction
- Position Embeddings 有用學習出來的(參考:https://zhuanlan.zhihu.com/p/46652512),也有用"Attention is all you need"中的三角函數算的(參考:https://github.com/codertimo/BERT-pytorch/blob/master/bert_pytorch/model/embedding/position.py)
另外之前以為輸入一定要是一個完整句子,後來發現我錯了:
Throughout this work, a “sentence” can be an arbitrary span of contiguous text, rather than an actual linguistic sentence.
A “sequence” refers to the input token sequence to BERT, which may be a single sentence or two sentences packed together.
Pre-training Tasks
unsupervised prediction tasks:
paper從這裡開始我就沒有看的很深入了......
- Masked LM
- Next Sentence Prediction
以上兩種pre-training 方法可以看論文比較快,或是參考這篇:https://daiwk.github.io/posts/nlp-bert.html
(How to) Fine-tuning
Experiment & Conclusion
比較特別的是paper中提到 BERT 也能用於 feature-based 中。
沒有留言:
張貼留言